try to reduce gpu_memory_utilization to some lower coefficient
Simeon Emanuilov PRO
s-emanuilov
AI & ML interests
Software Engineer & Ph.D. candidate | Specializing in ML/DL system development & applying AI to solve real-world business problems.
Recent Activity
replied to
their
post
about 3 hours ago
Tutorial ๐ฅ Training a non-English reasoning model with GRPO and Unsloth
I wanted to share my experiment with training reasoning models in languages other than English/Chinese.
Using Llama 3.1 8B as base, GRPO trainer from trl, and Unsloth optimizations, I got a working prototype in Bulgarian after ~5 hours on an L40S GPU. The approach should work for any language where the base model has some pre-training coverage.
Full code and tutorial here: https://unfoldai.com/reasoning-in-a-non-english-language/
The model itself: https://huggingface.co/s-emanuilov/LLMBG-Llama-3.1-8B-BG-Reasoning-v0.1
I hope this helps anyone looking to build reasoning models in their language.
upvoted
a
paper
1 day ago
Fast Video Generation with Sliding Tile Attention
replied to
their
post
2 days ago
Tutorial ๐ฅ Training a non-English reasoning model with GRPO and Unsloth
I wanted to share my experiment with training reasoning models in languages other than English/Chinese.
Using Llama 3.1 8B as base, GRPO trainer from trl, and Unsloth optimizations, I got a working prototype in Bulgarian after ~5 hours on an L40S GPU. The approach should work for any language where the base model has some pre-training coverage.
Full code and tutorial here: https://unfoldai.com/reasoning-in-a-non-english-language/
The model itself: https://huggingface.co/s-emanuilov/LLMBG-Llama-3.1-8B-BG-Reasoning-v0.1
I hope this helps anyone looking to build reasoning models in their language.
Organizations
s-emanuilov's activity
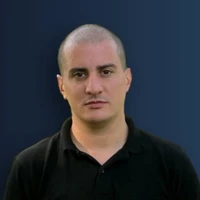
replied to
their
post
about 3 hours ago
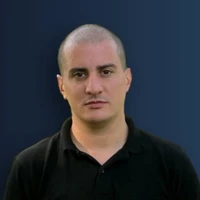
replied to
their
post
2 days ago
Thank you.
Iโm also a big fan of Qwen models. However, in this case, I donโt think they are appropriate because Iโm not entirely confident in their capabilities regarding multilingual contexts. Thatโs why I chose Llama.
Overall, I agree that the Qwen series is excellent for most tasks.
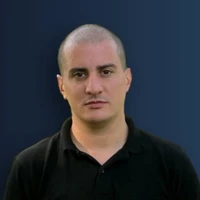
posted
an
update
2 days ago
Post
4794
Tutorial ๐ฅ Training a non-English reasoning model with GRPO and Unsloth
I wanted to share my experiment with training reasoning models in languages other than English/Chinese.
Using Llama 3.1 8B as base, GRPO trainer from trl, and Unsloth optimizations, I got a working prototype in Bulgarian after ~5 hours on an L40S GPU. The approach should work for any language where the base model has some pre-training coverage.
Full code and tutorial here: https://unfoldai.com/reasoning-in-a-non-english-language/
The model itself: s-emanuilov/LLMBG-Llama-3.1-8B-BG-Reasoning-v0.1
I hope this helps anyone looking to build reasoning models in their language.
I wanted to share my experiment with training reasoning models in languages other than English/Chinese.
Using Llama 3.1 8B as base, GRPO trainer from trl, and Unsloth optimizations, I got a working prototype in Bulgarian after ~5 hours on an L40S GPU. The approach should work for any language where the base model has some pre-training coverage.
Full code and tutorial here: https://unfoldai.com/reasoning-in-a-non-english-language/
The model itself: s-emanuilov/LLMBG-Llama-3.1-8B-BG-Reasoning-v0.1
I hope this helps anyone looking to build reasoning models in their language.
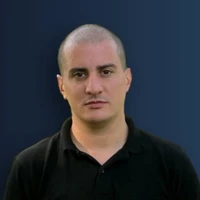
reacted to
m-ric's
post with ๐ฅ
6 days ago
Post
9173
Introducing ๐ผ๐ฝ๐ฒ๐ป ๐๐ฒ๐ฒ๐ฝ-๐ฅ๐ฒ๐๐ฒ๐ฎ๐ฟ๐ฐ๐ต by Hugging Face! ๐ฅ
OpenAI's latest agentic app Deep Research seems really good... But it's closed, as usual.
โฑ๏ธ So with a team of cracked colleagues, we set ourselves a 24hours deadline to replicate and open-source Deep Research! โฑ๏ธ
โก๏ธ We built open-Deep-Research, an entirely open agent that can: navigate the web autonomously, scroll and search through pages, download and manipulate files, run calculation on data...
We aimed for the best performance: are the agent's answers really rigorous?
On GAIA benchmark, Deep Research had 67% accuracy on the validation set.
โก๏ธ open Deep Research is at 55% (powered by o1), it is:
- the best pass@1 solution submitted
- the best open solution ๐ช๐ช
And it's only getting started ! Please jump in, drop PRs, and let's bring it to the top !
Read the blog post ๐ https://huggingface.co/blog/open-deep-research
OpenAI's latest agentic app Deep Research seems really good... But it's closed, as usual.
โฑ๏ธ So with a team of cracked colleagues, we set ourselves a 24hours deadline to replicate and open-source Deep Research! โฑ๏ธ
โก๏ธ We built open-Deep-Research, an entirely open agent that can: navigate the web autonomously, scroll and search through pages, download and manipulate files, run calculation on data...
We aimed for the best performance: are the agent's answers really rigorous?
On GAIA benchmark, Deep Research had 67% accuracy on the validation set.
โก๏ธ open Deep Research is at 55% (powered by o1), it is:
- the best pass@1 solution submitted
- the best open solution ๐ช๐ช
And it's only getting started ! Please jump in, drop PRs, and let's bring it to the top !
Read the blog post ๐ https://huggingface.co/blog/open-deep-research
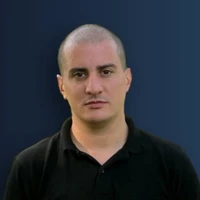
reacted to
AdinaY's
post with ๐ฅ
21 days ago
Post
2823
BIG release by DeepSeek AI๐ฅ๐ฅ๐ฅ
DeepSeek-R1 & DeepSeek-R1-Zero: two 660B reasoning models are here, alongside 6 distilled dense models (based on Llama & Qwen) for the community!
https://huggingface.co/deepseek-ai
deepseek-ai/DeepSeek-R1
โจ MIT License : enabling distillation for custom models
โจ 32B & 70B models match OpenAI o1-mini in multiple capabilities
โจ API live now! Access Chain of Thought reasoning with model='deepseek-reasoner'
DeepSeek-R1 & DeepSeek-R1-Zero: two 660B reasoning models are here, alongside 6 distilled dense models (based on Llama & Qwen) for the community!
https://huggingface.co/deepseek-ai
deepseek-ai/DeepSeek-R1
โจ MIT License : enabling distillation for custom models
โจ 32B & 70B models match OpenAI o1-mini in multiple capabilities
โจ API live now! Access Chain of Thought reasoning with model='deepseek-reasoner'
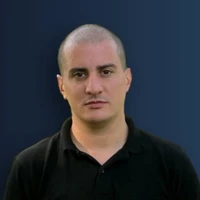
reacted to
merve's
post with โค๏ธ
25 days ago
Post
2571
Everything that happened this week in open AI, a recap ๐ค
merve/jan-17-releases-678a673a9de4a4675f215bf5
๐ Multimodal
- MiniCPM-o 2.6 is a new sota any-to-any model by OpenBMB
(vision, speech and text!)
- VideoChat-Flash-Qwen2.5-2B is new video multimodal models by OpenGVLab that come in sizes 2B & 7B in resolutions 224 & 448
- ByteDance released larger SA2VA that comes in 26B parameters
- Dataset: VRC-Bench is a new diverse benchmark for multimodal LLM reasoning performance
๐ฌ LLMs
- MiniMax-Text-01 is a new huge language model (456B passive 45.9B active params) by MiniMaxAI with context length of 4M tokens ๐คฏ
- Dataset: Sky-T1-data-17k is a diverse dataset used to train Sky-T1-32B
- kyutai released Helium-1-Preview-2B is a new small multilingual LM
- Wayfarer-12B is a new LLM able to write D&D ๐ง๐ปโโ๏ธ
- ReaderLM-v2 is a new HTML parsing model by Jina AI
- Dria released, Dria-Agent-a-3B, new agentic coding model (Pythonic function calling) based on Qwen2.5 Coder
- Unsloth released Phi-4, faster and memory efficient Llama 3.3
๐ผ๏ธ Vision
- MatchAnything is a new foundation model for matching
- FitDit is a high-fidelity VTON model based on DiT architecture
๐ฃ๏ธ Audio
- OuteTTS-0.3-1B is a new multilingual text-to-speech model with voice cloning and emotion control capabilities
๐ Retrieval
- lightblue released a new reranker based on Qwen2.5 LB-reranker-0.5B-v1.0 that can handle 95+ languages
- cde-small-v2 is a new sota small retrieval model by
@jxm
๐ Multimodal
- MiniCPM-o 2.6 is a new sota any-to-any model by OpenBMB
(vision, speech and text!)
- VideoChat-Flash-Qwen2.5-2B is new video multimodal models by OpenGVLab that come in sizes 2B & 7B in resolutions 224 & 448
- ByteDance released larger SA2VA that comes in 26B parameters
- Dataset: VRC-Bench is a new diverse benchmark for multimodal LLM reasoning performance
๐ฌ LLMs
- MiniMax-Text-01 is a new huge language model (456B passive 45.9B active params) by MiniMaxAI with context length of 4M tokens ๐คฏ
- Dataset: Sky-T1-data-17k is a diverse dataset used to train Sky-T1-32B
- kyutai released Helium-1-Preview-2B is a new small multilingual LM
- Wayfarer-12B is a new LLM able to write D&D ๐ง๐ปโโ๏ธ
- ReaderLM-v2 is a new HTML parsing model by Jina AI
- Dria released, Dria-Agent-a-3B, new agentic coding model (Pythonic function calling) based on Qwen2.5 Coder
- Unsloth released Phi-4, faster and memory efficient Llama 3.3
๐ผ๏ธ Vision
- MatchAnything is a new foundation model for matching
- FitDit is a high-fidelity VTON model based on DiT architecture
๐ฃ๏ธ Audio
- OuteTTS-0.3-1B is a new multilingual text-to-speech model with voice cloning and emotion control capabilities
๐ Retrieval
- lightblue released a new reranker based on Qwen2.5 LB-reranker-0.5B-v1.0 that can handle 95+ languages
- cde-small-v2 is a new sota small retrieval model by
@jxm
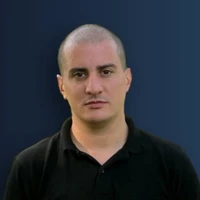
reacted to
tomaarsen's
post with โค๏ธ
26 days ago
Post
4581
๐๏ธ Today I'm introducing a method to train static embedding models that run 100x to 400x faster on CPU than common embedding models, while retaining 85%+ of the quality! Including 2 fully open models: training scripts, datasets, metrics.
We apply our recipe to train 2 Static Embedding models that we release today! We release:
2๏ธโฃ an English Retrieval model and a general-purpose Multilingual similarity model (e.g. classification, clustering, etc.), both Apache 2.0
๐ง my modern training strategy: ideation -> dataset choice -> implementation -> evaluation
๐ my training scripts, using the Sentence Transformers library
๐ my Weights & Biases reports with losses & metrics
๐ my list of 30 training and 13 evaluation datasets
The 2 Static Embedding models have the following properties:
๐๏ธ Extremely fast, e.g. 107500 sentences per second on a consumer CPU, compared to 270 for 'all-mpnet-base-v2' and 56 for 'gte-large-en-v1.5'
0๏ธโฃ Zero active parameters: No Transformer blocks, no attention, not even a matrix multiplication. Super speed!
๐ No maximum sequence length! Embed texts at any length (note: longer texts may embed worse)
๐ Linear instead of exponential complexity: 2x longer text takes 2x longer, instead of 2.5x or more.
๐ช Matryoshka support: allow you to truncate embeddings with minimal performance loss (e.g. 4x smaller with a 0.56% perf. decrease for English Similarity tasks)
Check out the full blogpost if you'd like to 1) use these lightning-fast models or 2) learn how to train them with consumer-level hardware: https://huggingface.co/blog/static-embeddings
The blogpost contains a lengthy list of possible advancements; I'm very confident that our 2 models are only the tip of the iceberg, and we may be able to get even better performance.
Alternatively, check out the models:
* sentence-transformers/static-retrieval-mrl-en-v1
* sentence-transformers/static-similarity-mrl-multilingual-v1
We apply our recipe to train 2 Static Embedding models that we release today! We release:
2๏ธโฃ an English Retrieval model and a general-purpose Multilingual similarity model (e.g. classification, clustering, etc.), both Apache 2.0
๐ง my modern training strategy: ideation -> dataset choice -> implementation -> evaluation
๐ my training scripts, using the Sentence Transformers library
๐ my Weights & Biases reports with losses & metrics
๐ my list of 30 training and 13 evaluation datasets
The 2 Static Embedding models have the following properties:
๐๏ธ Extremely fast, e.g. 107500 sentences per second on a consumer CPU, compared to 270 for 'all-mpnet-base-v2' and 56 for 'gte-large-en-v1.5'
0๏ธโฃ Zero active parameters: No Transformer blocks, no attention, not even a matrix multiplication. Super speed!
๐ No maximum sequence length! Embed texts at any length (note: longer texts may embed worse)
๐ Linear instead of exponential complexity: 2x longer text takes 2x longer, instead of 2.5x or more.
๐ช Matryoshka support: allow you to truncate embeddings with minimal performance loss (e.g. 4x smaller with a 0.56% perf. decrease for English Similarity tasks)
Check out the full blogpost if you'd like to 1) use these lightning-fast models or 2) learn how to train them with consumer-level hardware: https://huggingface.co/blog/static-embeddings
The blogpost contains a lengthy list of possible advancements; I'm very confident that our 2 models are only the tip of the iceberg, and we may be able to get even better performance.
Alternatively, check out the models:
* sentence-transformers/static-retrieval-mrl-en-v1
* sentence-transformers/static-similarity-mrl-multilingual-v1
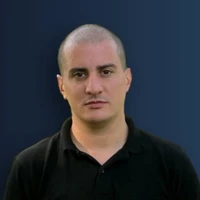
posted
an
update
27 days ago
Post
495
A new benchmark (DPAB-ฮฑ) has been released that evaluates LLM function calling in both Pythonic and JSON approaches.
It shows that Pythonic function calling often outperforms traditional JSON-based methods, especially for complex multi-step tasks.
Key findings from benchmarks:
โ Claude 3.5 Sonnet leads with 87% on Pythonic vs 45% on JSON
โ Smaller models show impressive results (Dria-Agent-ฮฑ-3B: 72% Pythonic)
โ Even larger models like DeepSeek V3 (685B) show significant gaps (63% Pythonic vs 33% JSON)
If you're building or using LLM agents, these results suggest that how you implement function calling could impact performance - might be worth reconsidering JSON-only approaches.
The benchmark: https://github.com/firstbatchxyz/function-calling-eval
Blog post: https://huggingface.co/blog/andthattoo/dpab-a
It shows that Pythonic function calling often outperforms traditional JSON-based methods, especially for complex multi-step tasks.
Key findings from benchmarks:
โ Claude 3.5 Sonnet leads with 87% on Pythonic vs 45% on JSON
โ Smaller models show impressive results (Dria-Agent-ฮฑ-3B: 72% Pythonic)
โ Even larger models like DeepSeek V3 (685B) show significant gaps (63% Pythonic vs 33% JSON)
If you're building or using LLM agents, these results suggest that how you implement function calling could impact performance - might be worth reconsidering JSON-only approaches.
The benchmark: https://github.com/firstbatchxyz/function-calling-eval
Blog post: https://huggingface.co/blog/andthattoo/dpab-a
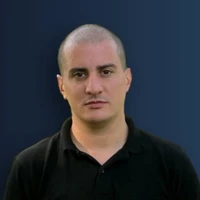
reacted to
AdinaY's
post with ๐ฅ
27 days ago
Post
3108
MiniMax, the company behind Hailuo_AI, has joined the open source community by releasing both models and demos of MiniMax-Text-01 & MiniMax-VL-01๐ฅ
- Model
MiniMaxAI/MiniMax-VL-01
MiniMaxAI/MiniMax-Text-01
- Demo
MiniMaxAI/MiniMax-VL-01
MiniMaxAI/MiniMax-Text-01
โจ MiniMax-text-01:
- 456B with 45.9B activated per token
- Combines Lightning Attention, Softmax Attention, and MoE for optimal performance
- Training context up to 1M tokens, inference handles 4M tokens
โจ MiniMax-VL-01:
- ViT-MLP-LLM framework ( non-transformer๐)
- Handles image inputs from 336ร336 to 2016ร2016
- 694M image-caption pairs + 512B tokens processed across 4 stages
- Model
MiniMaxAI/MiniMax-VL-01
MiniMaxAI/MiniMax-Text-01
- Demo
MiniMaxAI/MiniMax-VL-01
MiniMaxAI/MiniMax-Text-01
โจ MiniMax-text-01:
- 456B with 45.9B activated per token
- Combines Lightning Attention, Softmax Attention, and MoE for optimal performance
- Training context up to 1M tokens, inference handles 4M tokens
โจ MiniMax-VL-01:
- ViT-MLP-LLM framework ( non-transformer๐)
- Handles image inputs from 336ร336 to 2016ร2016
- 694M image-caption pairs + 512B tokens processed across 4 stages
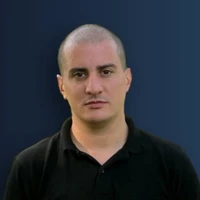
posted
an
update
30 days ago
Post
556
New paper from Salesforce AI Research. The authors found that joint training, continual pre-training (CPT), and instruction tuning with a 50/50 data split achieve better results than sequential training. Their 8B parameter model outperformed larger 70B models on financial tasks.
Down-sampling CPT data to match IT data size improved performance on CFA Challenge exams from 34.44% to 55.56%, while maintaining strong general knowledge capabilities as shown by comparable or better performance on general knowledge benchmarks like AI2-ARC and MMLU.
Technical implementation involved two-stage training: Group 1 utilized 3.84B tokens from web and basic texts, followed by Group 2, which used 1.66B tokens from domain-specific books. Their preference alignment method used generative reward models to identify and correct reasoning errors rather than just rating full solutions.
Evaluation on 91,872 samples across 31 tasks showed their Llama-Fin model achieving 91.13% accuracy on sentiment analysis (FPB) and 95.32% on FiQA SA, exceeding GPT-4's performance of 82.16% and 68.51%, respectively, on these benchmarks.
It could be useful for many financial companies looking to build AI pipelines.
Interesting read, but neither the model nor GitHub repo is accessible yet. The key insight for AI builders is that with small models - it is fully possible to outperform much bigger models.
https://arxiv.org/abs/2501.04961
Down-sampling CPT data to match IT data size improved performance on CFA Challenge exams from 34.44% to 55.56%, while maintaining strong general knowledge capabilities as shown by comparable or better performance on general knowledge benchmarks like AI2-ARC and MMLU.
Technical implementation involved two-stage training: Group 1 utilized 3.84B tokens from web and basic texts, followed by Group 2, which used 1.66B tokens from domain-specific books. Their preference alignment method used generative reward models to identify and correct reasoning errors rather than just rating full solutions.
Evaluation on 91,872 samples across 31 tasks showed their Llama-Fin model achieving 91.13% accuracy on sentiment analysis (FPB) and 95.32% on FiQA SA, exceeding GPT-4's performance of 82.16% and 68.51%, respectively, on these benchmarks.
It could be useful for many financial companies looking to build AI pipelines.
Interesting read, but neither the model nor GitHub repo is accessible yet. The key insight for AI builders is that with small models - it is fully possible to outperform much bigger models.
https://arxiv.org/abs/2501.04961
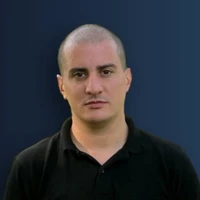
reacted to
danielhanchen's
post with ๐ฅ
about 1 month ago
Post
4644
We fixed many bugs in Phi-4 & uploaded fixed GGUF + 4-bit versions! โจ
Our fixed versions are even higher on the Open LLM Leaderboard than Microsoft's!
GGUFs: unsloth/phi-4-GGUF
Dynamic 4-bit: unsloth/phi-4-unsloth-bnb-4bit
You can also now finetune Phi-4 for free on Colab: https://colab.research.google.com/github/unslothai/notebooks/blob/main/nb/Phi_4-Conversational.ipynb
Read our blogpost for more details on bug fixes etc: https://unsloth.ai/blog/phi4
Our fixed versions are even higher on the Open LLM Leaderboard than Microsoft's!
GGUFs: unsloth/phi-4-GGUF
Dynamic 4-bit: unsloth/phi-4-unsloth-bnb-4bit
You can also now finetune Phi-4 for free on Colab: https://colab.research.google.com/github/unslothai/notebooks/blob/main/nb/Phi_4-Conversational.ipynb
Read our blogpost for more details on bug fixes etc: https://unsloth.ai/blog/phi4
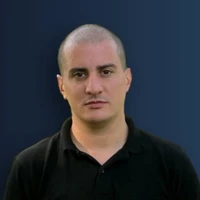
replied to
their
post
about 1 month ago
Yeah, the issues with the tables.
For office formats, it's mostly fine. You tried using PDF or images?
I will work on improving this.
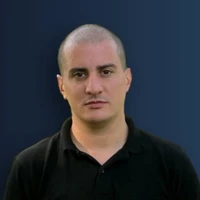
replied to
their
post
about 1 month ago
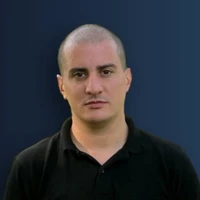
reacted to
merve's
post with ๐ฅ
about 1 month ago
Post
4856
supercharge your LLM apps with smolagents ๐ฅ
however cool your LLM is, without being agentic it can only go so far
enter smolagents: a new agent library by Hugging Face to make the LLM write code, do analysis and automate boring stuff!
Here's our blog for you to get started https://huggingface.co/blog/smolagents
however cool your LLM is, without being agentic it can only go so far
enter smolagents: a new agent library by Hugging Face to make the LLM write code, do analysis and automate boring stuff!
Here's our blog for you to get started https://huggingface.co/blog/smolagents
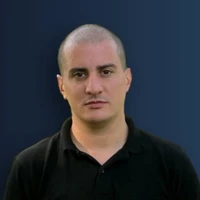
posted
an
update
about 1 month ago
Post
2577
Hey HF community! ๐
Excited to share Monkt - a tool I built to solve the eternal headache of processing documents for ML/AI pipelines.
What it does: Converts PDFs, Word, PowerPoint, Excel, Web pages or raw HTML into clean Markdown or structured JSON.
Great for:
โ LLM training dataset preparation;
โ Knowledge base construction;
โ Research paper processing;
โ Technical documentation management.
It has API access for integration into ML pipelines.
Check it out at https://monkt.com/ if you want to save time on document processing infrastructure.
Looking forward to your feedback!
Excited to share Monkt - a tool I built to solve the eternal headache of processing documents for ML/AI pipelines.
What it does: Converts PDFs, Word, PowerPoint, Excel, Web pages or raw HTML into clean Markdown or structured JSON.
Great for:
โ LLM training dataset preparation;
โ Knowledge base construction;
โ Research paper processing;
โ Technical documentation management.
It has API access for integration into ML pipelines.
Check it out at https://monkt.com/ if you want to save time on document processing infrastructure.
Looking forward to your feedback!